引用本文: | 王怡,张勇,周岳,刘卉昆,李倩,李楠楠,王潇,张宁宁,朱崇抒.2025.西安北部区域夏季PM2.5化学组分昼夜变化特征及来源解析:在线观测应用案例[J].地球环境学报,16(2):247-256 |
| WANG Yi,ZHANG Yong,ZHOU Yue,LIU Huikun,LI Qian,LI Nannan,WANG Xiao,ZHANG Ningning,ZHU Chongshu.2025.Diurnal variation characteristics and source analysis of PM2.5 chemical components in summer in the northern region of Xi’an: a case study of online observation applications[J].Journal of Earth Environment,16(2):247-256 |
|
|
|
本文已被:浏览 0次 下载 0次 |
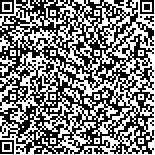 码上扫一扫! |
|
西安北部区域夏季PM2.5化学组分昼夜变化特征及来源解析:在线观测应用案例 |
王怡1, 2,张勇1, 2,周岳1,刘卉昆1,李倩3,李楠楠3,王潇3,张宁宁1*,朱崇抒1
|
1. 中国科学院地球环境研究所 中国科学院气溶胶化学与物理重点实验室,西安 710061
2. 中国科学院大学,北京 100049
3. 西安地球环境创新研究院,西安 710061
|
|
摘要: |
为探究西安北部区域大气PM2.5化学组成及其来源变化特征,于2020年夏季利用实时在线大气污染移动监测平台,监测大气PM2.5中有机碳(OC)、元素碳(EC)、水溶性离子及无机元素等组分,并采用CAS-HERM来源解析模型获得其来源。结果表明:观测期间西安北部区域夏季PM2.5浓度夜间高于白天,其中OC和EC组分浓度夜间分别较白天高2.4 μg∙m−3和0.5 μg∙m−3,元素Al、Si、Ca、K和Fe也均呈现明显夜高昼低的变化特征。水溶性离子中超过1/2的离子夜间质量浓度高于白天,SO42−、NO3−和NH4+是主要的水溶性离子,三者对总离子浓度的贡献白天(81.8%)高于夜间(75.2%)。元素富集因子结果表明Al、Cu、As、Zn、Pb、Sn、Hg、Se和Cd受人为活动影响明显。CAS-HERM源解析结果表明PM2.5污染源主要包括机动车源、燃煤源及扬尘源,并叠加二次生成污染物的影响,其中一次源对PM2.5的日均贡献较二次源高25.7%,且一次源夜间贡献(64.2%)高于白天(61.5%)。 |
关键词: PM2.5 化学组分 来源解析 在线观测 |
DOI:10.7515/JEE232036 |
CSTR:32259.14.JEE232036 |
分类号: |
基金项目:中国科学院“西部之光”人才培养计划(XAB2021YN06) |
英文基金项目:CAS “Light of West China” Program (XAB2021YN06) |
|
Diurnal variation characteristics and source analysis of PM2.5 chemical components in summer in the northern region of Xi’an: a case study of online observation applications |
WANG Yi1, 2, ZHANG Yong1, 2, ZHOU Yue1, LIU Huikun1, LI Qian3, LI Nannan3, WANG Xiao3, ZHANG Ningning1*, ZHU Chongshu1
|
1. Key Laboratory of Aerosol Chemistry and Physics, Institute of Earth Environment, Chinese Academy of Sciences, Xi’an 710061, China
2. University of Chinese Academy of Sciences, Beijing 100049, China
3. Xi’an Institute for Innovative Earth Environment Research, Xi’an 710061, China
|
Abstract: |
Background, aim, and scope Fine particulate matter (PM2.5) is a major concern due to its serious impact on human health and air quality. Xi’an, a typical northern Chinese city, is experiencing severe air pollution. It is critical to further improve air quality by understanding the diurnal variations of chemical components and the source contribution of PM2.5. This study focuses on that during the summer and aims to provide scientific insights into the prevention and management of air pollution in Xi’an. Materials and methods A real-time campaign for PM2.5 was conducted from August 20th to September 4th, 2020, at Gaoling District in Xi’an. The PM2.5 and its chemical components, including carbonaceous aerosol, water-soluble ions, and trace elements, were monitored by an ambient air particulate matter automatic monitor (XHPM-2000E), an atmospheric aerosol elemental carbon/organic carbon analyzer (Sunset-OCEC(RT-4)), in-situ gas and aerosol composition monitor (S611-C), and an atmospheric heavy metal on-line monitor (XHAM-2000A), respectively. A hybrid environment receptor model (HERM) was used to resolve the sources contribution of PM2.5 during the campaign. Results The average concentration of PM2.5 during the campaign was (24.4±16.0) μg·m−3, and the concentration in daytime ((20.3±11.7) μg·m−3) was lower than that in nighttime ((28.5±18.5) μg·m−3). The concentrations of OC and EC in daytime were significantly lower than those in nighttime. However, the total average concentrations of water-soluble ions in daytime were basically higher than that in nighttime. SO42−, NO3− and NH4+ were the dominant components for total water-soluble ions, which all contributed 81.8% and 75.2% in daytime and nighttime, respectively. The total concentration of trace elements at night was higher than that during the daytime. Eight sources of PM2.5 were resolved by HERM, and the dominant sources during the daytime were secondary sulfate and motor vehicle emission, which contributed 20.6% and 17.6% to PM2.5, respectively. In contrast, the dominant sources of PM2.5 during the nighttime changed to motor vehicle emission and biomass burning, which contributed 19.8% and 13.9% to PM2.5, respectively. Discussion The average concentration of PM2.5 varied significantly from daytime to nighttime because of the low temperature, the high humidity, and the low-speed wind during the nighttime, leading to the generation and accumulation of pollutants. Additionally, with the increasing emission from heavy diesel vehicles at night, the concentration of EC intensity rose, resulting in the continuous increase of PM2.5 at night. At the same time, higher concentrations of metal elements at night were attributed to the increasing emissions from muck truck and biomass burning. The concentration of secondary inorganic ions was slightly higher during the daytime than at night. It was attributed to the high radiation, relatively high temperature, and humidity during the daytime, which is more conducive to the heterogeneous reaction. The primary source of pollutants mainly originated from local emissions, while the secondary source mainly emerged from the formation of homogeneous and heterogeneous reactions with nitrogen oxides and volatile organic compounds in the atmosphere. Conclusions The average concentration of PM2.5 at Gaoling was lower than the national ambient air quality standard, yet higher than the average level of Xi’an. And the concentration of PM2.5 was higher during the nighttime. Lower concentrations of carbon and trace elements were detected during daytime compared to nighttime. The concentrations of water-soluble ions were higher during the daytime than those at night. The contribution of primary emission sources at night was higher. Recommendations and perspectives This study provided a scientific understanding for the prevention and control of air pollution in Xi’an by investigating the diurnal variations of chemical composition and source contribution in PM2.5 during the summer. In the future, it is necessary to conduct online investigations on the spatial and temporal distribution, characteristics, formation mechanism, and source of PM2.5. |
Key words: PM2.5 chemical composition source apportionment online observation |
|
|
|
|
|